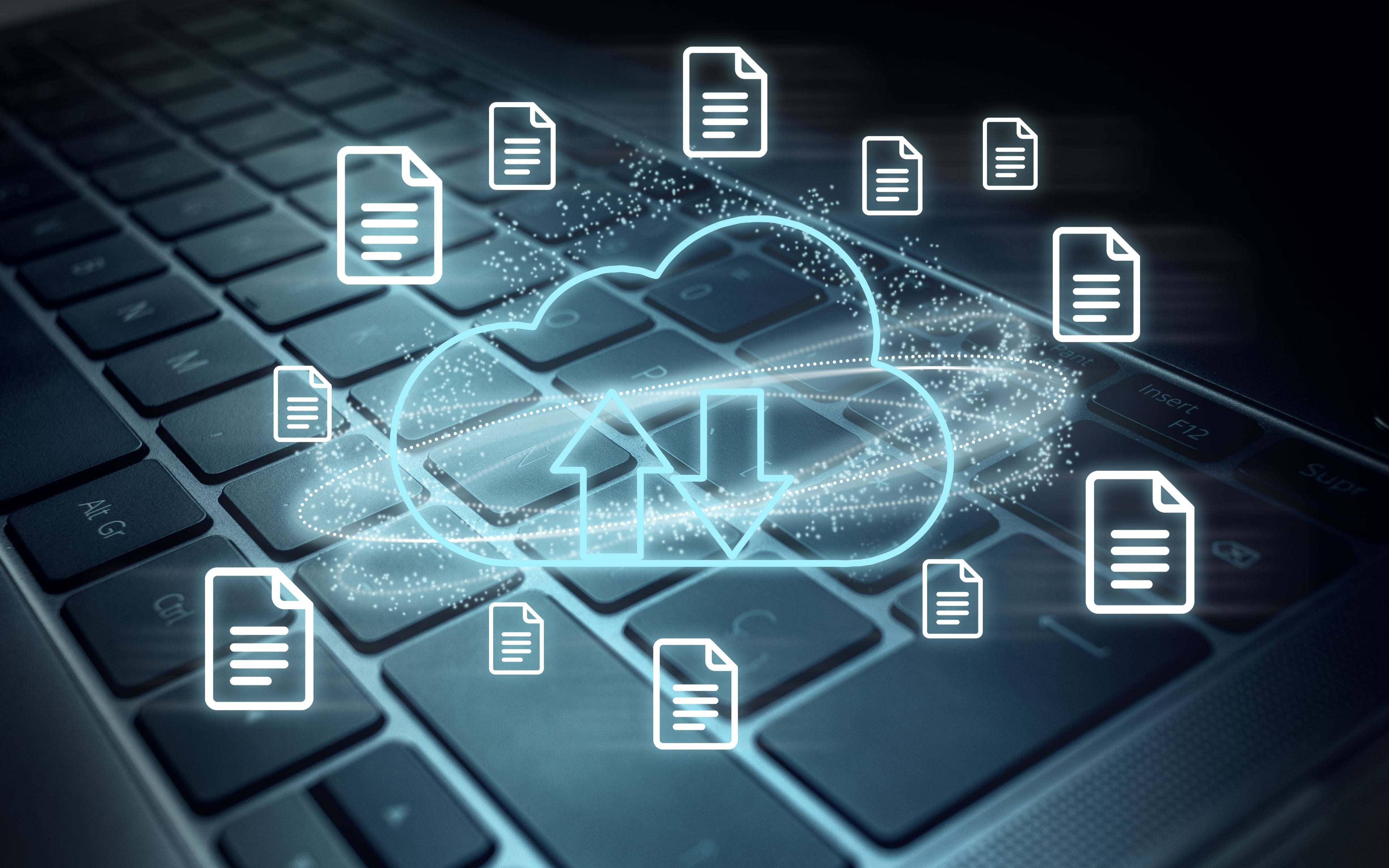
Snowflake Data Quality: Ensuring Accuracy in Snowflake data quality
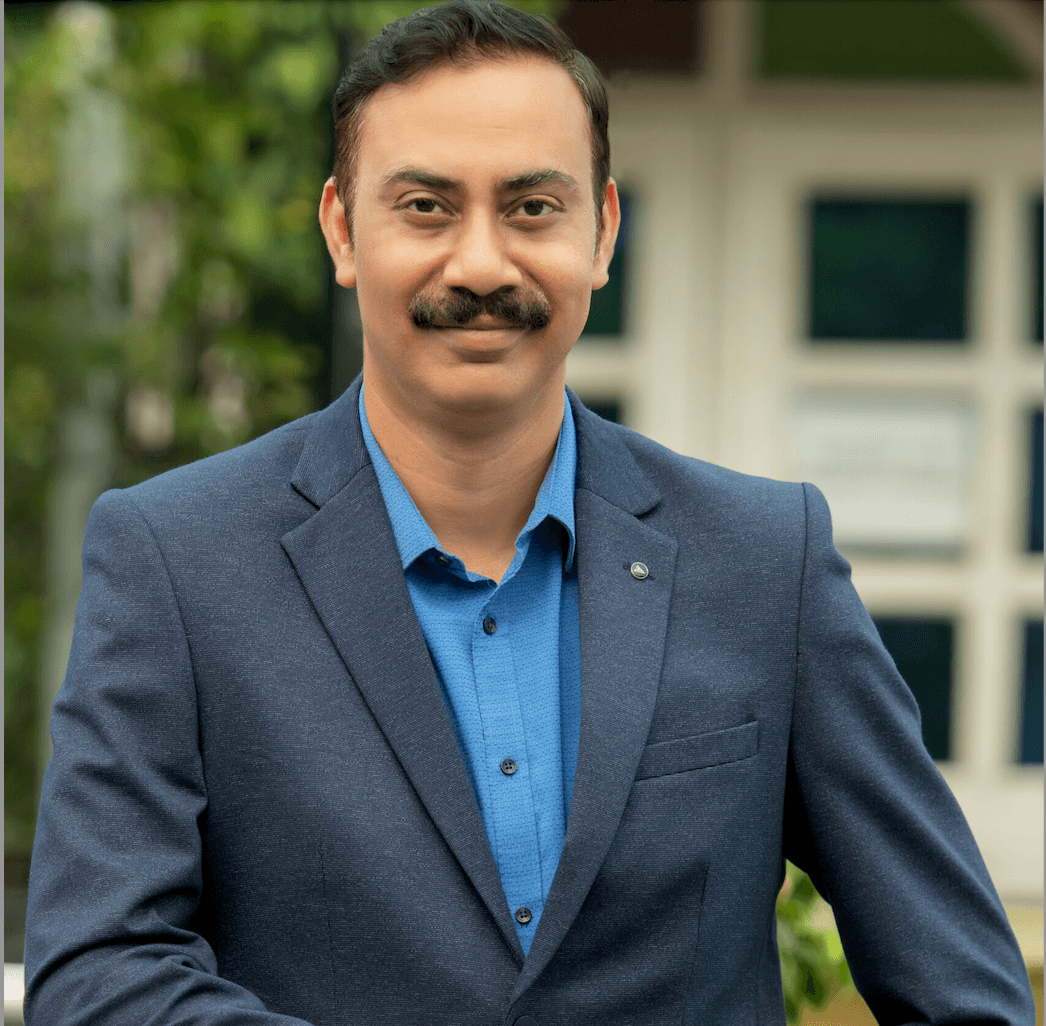
Snowflake Data Quality: Ensuring Accuracy in Snowflake data quality
In today’s data-driven landscape, maintaining top data quality standards is paramount for businesses aiming to leverage their data assets effectively. Snowflake, a leading cloud data warehouse, offers robust tools and features to ensure high-quality data, enabling organizations to make informed decisions and drive digital transformation.
Data quality refers to the accuracy, completeness, reliability, and relevance of data. In Snowflake, data quality monitoring is facilitated through various features and functions, including data metric functions, data profiling, data quality queries, and object tagging. Data profiling and data quality assessment are integral parts of this process, helping to identify and rectify data quality issues proactively. These data quality workflow tools help data teams identify and address data quality issues, such as null values, duplicate values, and outdated data.
Snowflake’s Access History feature provides valuable insights into data usage, helping track sensitive data and ensuring compliance with data governance frameworks. By using data lineage and analyzing access patterns, data engineers can monitor higher-risk data and configure alerts for any anomalies.
Data validation and testing are crucial components of maintaining data quality. Snowflake supports data quality rules and tests, allowing teams to validate data against predefined criteria. This data metric function includes checking for acceptable values, monitoring data freshness, and ensuring data integrity through schema tests and custom tests.
Object tagging in Snowflake aids in data classification and enhances data discovery. By consistently tagging objects, data governance teams can apply dynamic data masking and row-level access policies, safeguarding sensitive data and improving data observability.
Snowsight, Snowflake’s metadata-driven analytics interface, offers data visualizations and metadata insights, enabling more robust discovery and exploration of data assets. With features like frequency distributions, key distributions, and histograms, teams can gain valuable insights into data quality measurements and make data-driven decisions to maintain data quality.
In summary, Snowflake’s comprehensive suite of data quality tools and features empowers organizations to maintain high-quality data, ensuring data
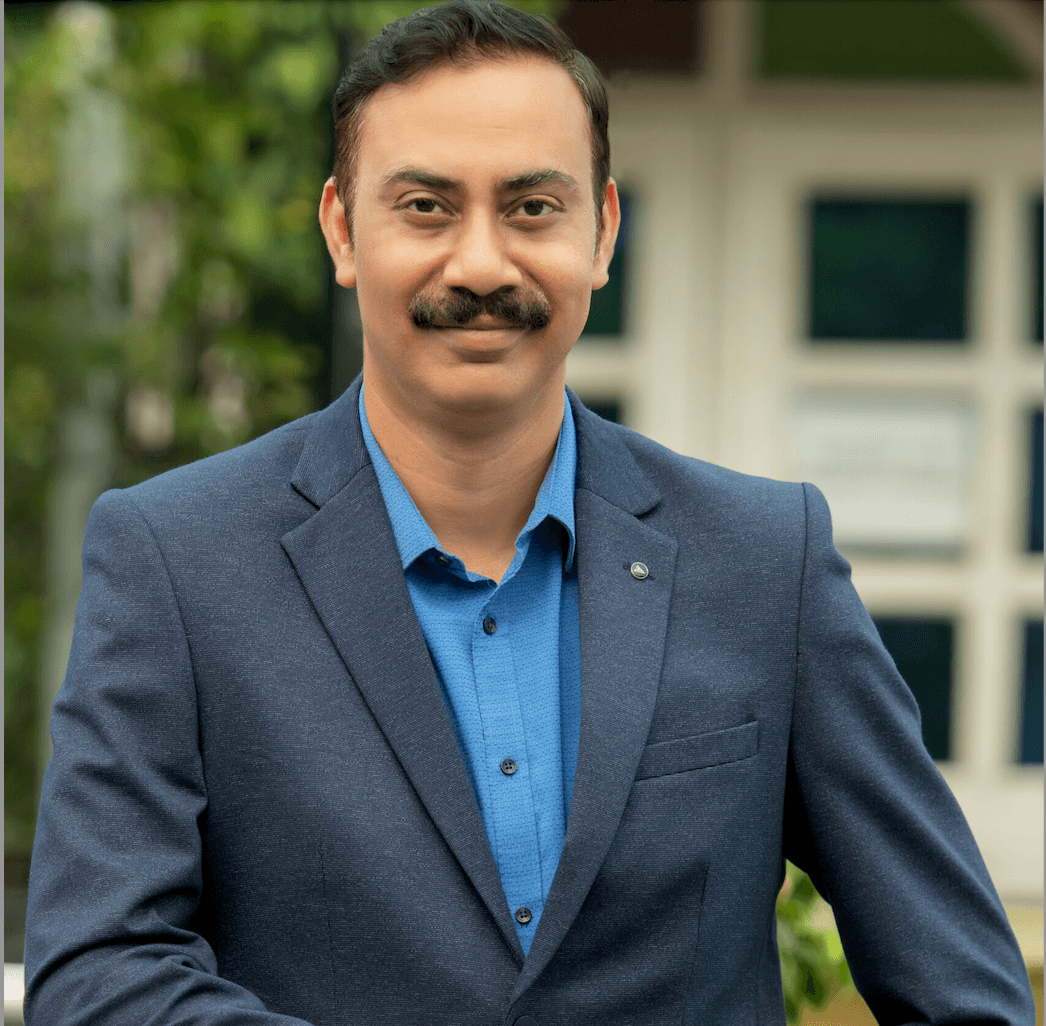
About Pronam Chatterjee
A visionary with 25 years of technical leadership under his belt, Pronam isn’t just ahead of the curve; he’s redefining it. His expertise extends beyond the technical, making him a sought-after speaker and published thought leader. Whether strategizing the next technology and data innovation or his next chess move, Pronam thrives on pushing boundaries. He is a father of two loving daughters and a Golden Retriever. With a blend of brilliance, vision, and genuine connection, Pronam is more than a leader; he’s an architect of the future, building something extraordinary
Related Posts
Master data management (MDM) is a process that enables organizations to define and manage the common data entities used across the enterprise.
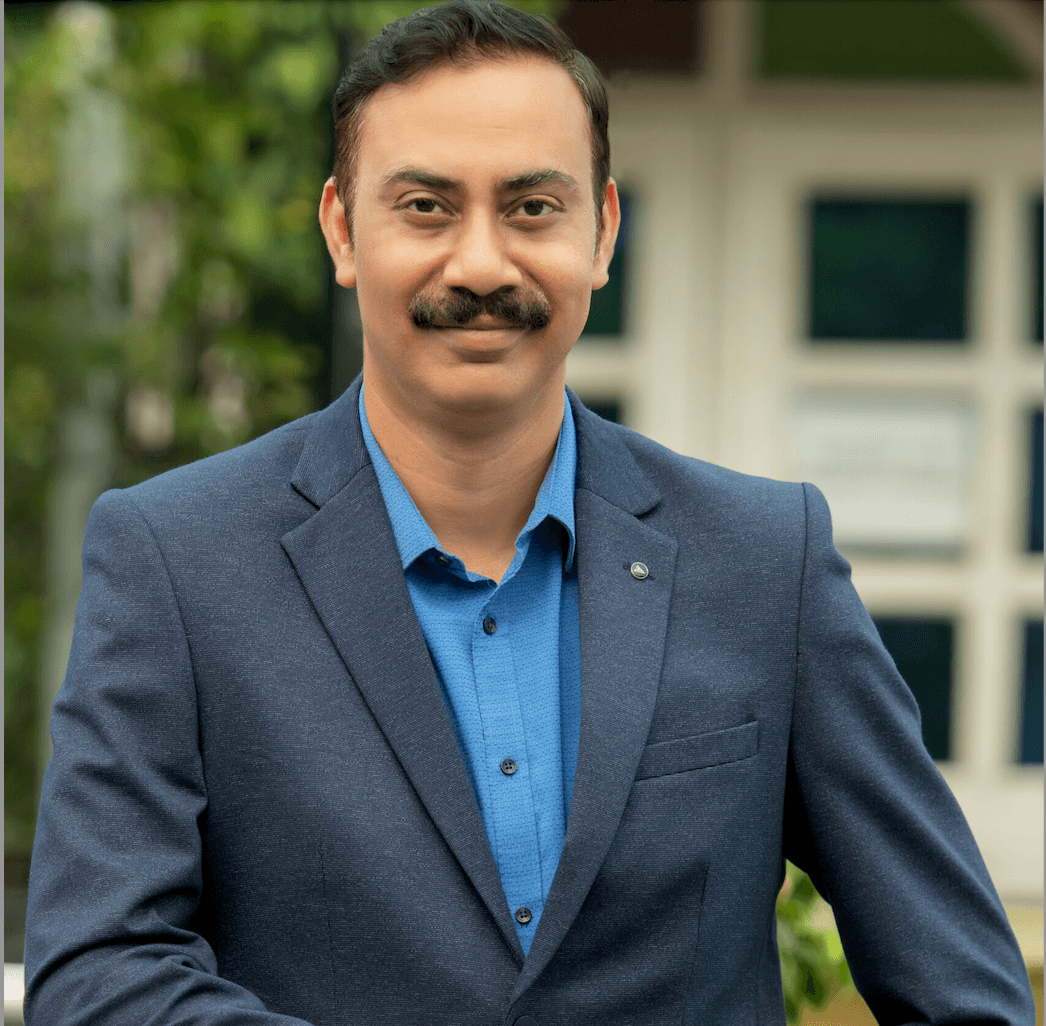
Data engineering involves designing systems to collect, store, and analyze data efficiently.
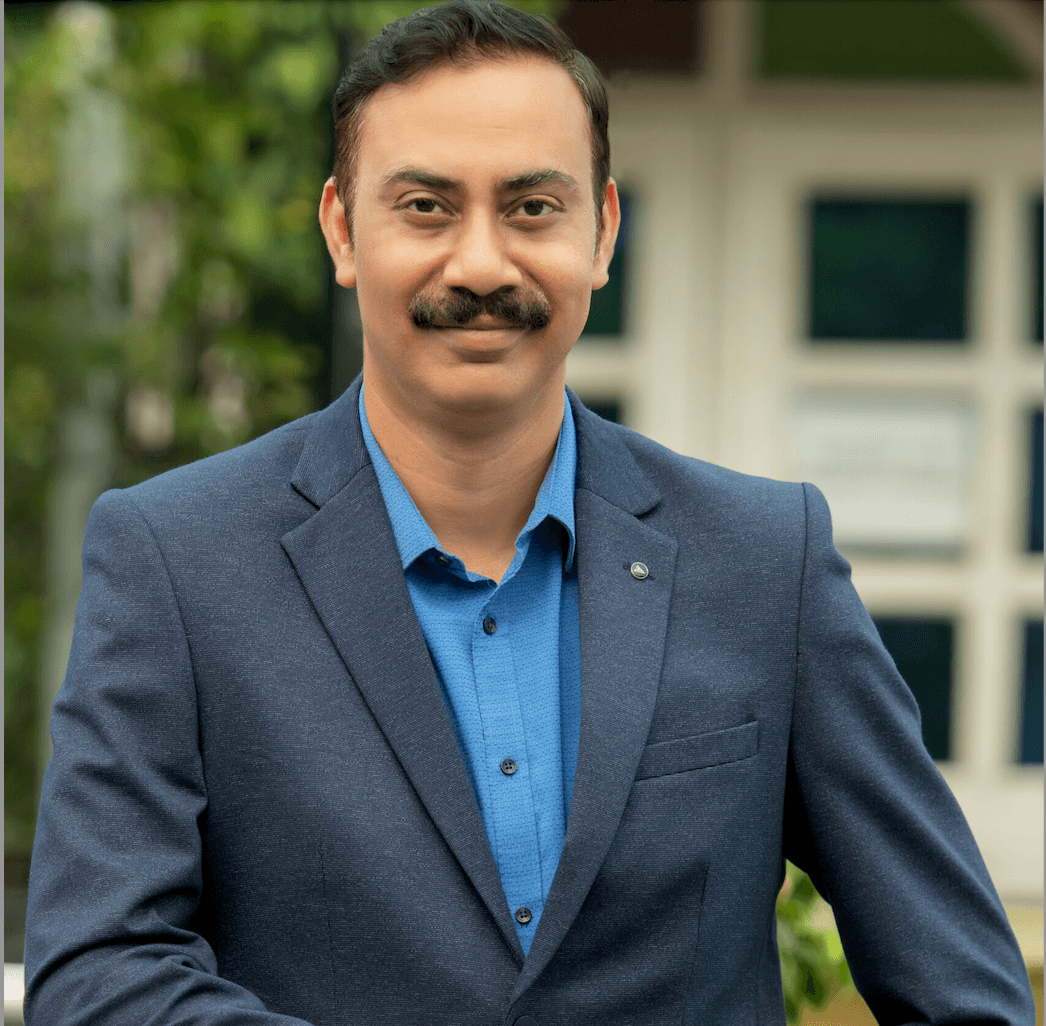
Cloud scalability, enables businesses to meet expected demand of business services without the need for large, up-front investments in infrastructure.
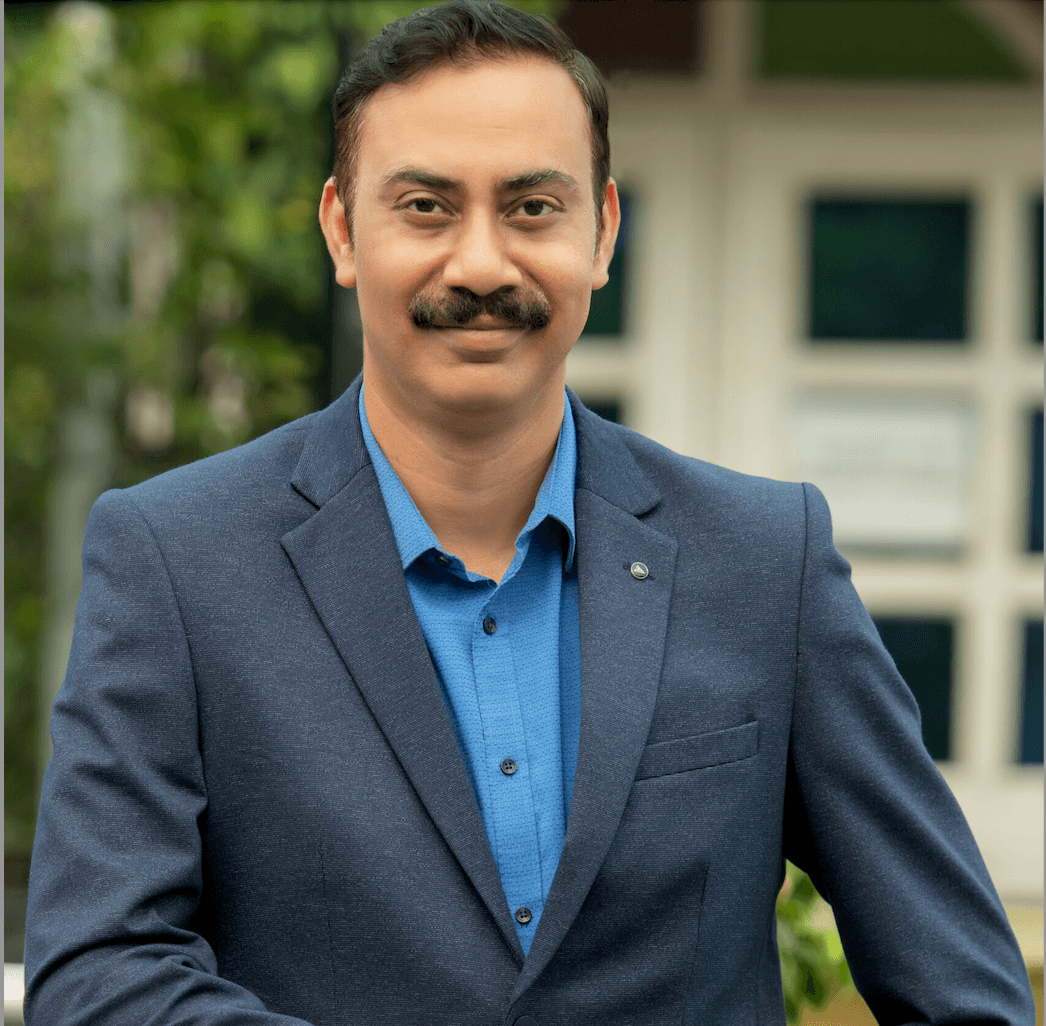