
Star Schema vs Snowflake Schema: find out the Warehouse model that is right for you
26 April 2024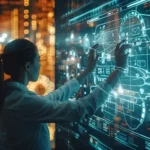
Breakthrough Data Architecture: 6 Reasons to Love Snowflake’s Unique Approach to Data Warehousing
10 May 2024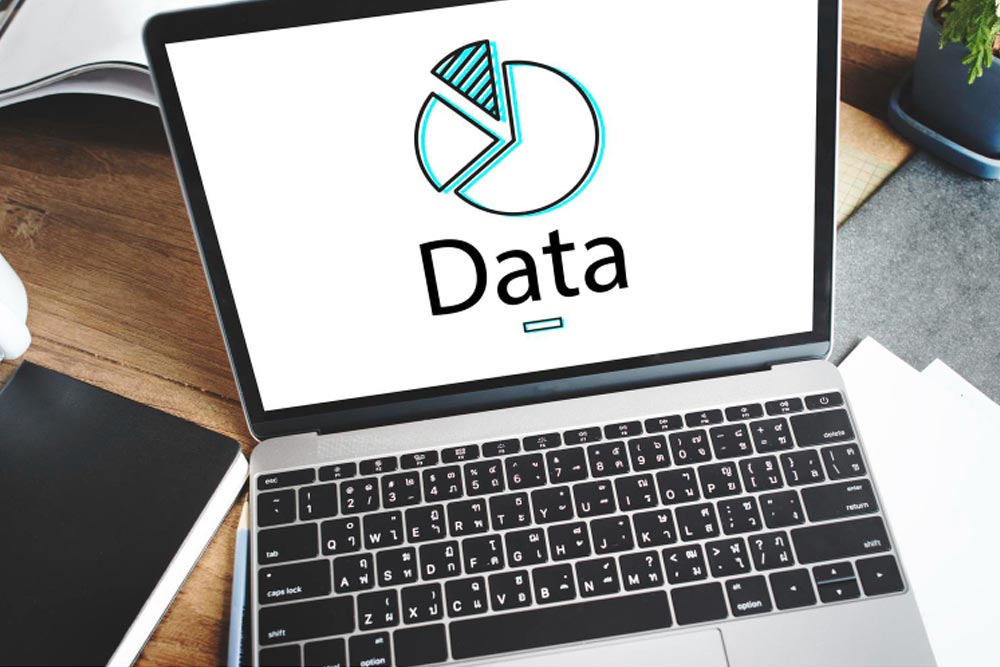
Tags

Published by
BluePi
Data-Driven Business Transformation
Unlock The Power of Your Data: Data Lake vs. Data Warehouse for Smarter Business Decisions (2024)
Introductory paragraph: Storage and analytics
Information sources are an imitation of today’ data driven period, when organizations are inundated with a vast amount of data. With the rising recognition of how data can give an advantage and proper decision making, effective data storage and management have been tagged as a lifeline for businesses to remain competitive.
Two prominent strategies have emerged: data lakes, and data warehousing. While they are used for the same purposes—that is, data storage and analysis—they differ from each other in terms of architecture, functions, and cases of use.
In the advent of 2024, we need an insight into these methods in order to reveal the potency of your data.
Explainable Data Repositories
A data lake is an assortment of data generated with the purpose of said raw data be put in its native, both structured and unstructured, format. Instead of conventional data warehouses, data lakes do not necessitate the preprocessing and transformation of excellent data processing, and offer greater flexibility and scalability.
Key characteristics of data lakes:
Schema-on-Read:
Data feeds into this structure in its raw condition, while the schema responsible for its querying and analysis is applied in the last stage when needed, thus allowing some flexibility in dealing with different data types within the system.
Cost-Effective Storage:
The use of commodity object storage that is affordable, such as Amazon S3 or Azure Data Lake Storage, to store big data in data lakes makes them very suitable.
Scalability:
Data lakes are simply known to accommodate the rising data volume without significant capacity degradation due to their easy scalability.
Advanced Analytics:
The retrieval capacity of data lakes is not limited to specific data types since they can support several forms of data, such as numeric, text, images, audio, and video formats, which are necessary for advanced analytics techniques like machine learning and artificial intelligence.
Pros:
- Storing and deciphering different types of data can become flexible.
- Online, our access to genealogical records, censuses, and military databases is unlimited, and there is always a new platform to explore.
- Cost-effective storage solutions
- Enabling state-of-art analytics solutions.
Cons:
- Possibility of poor data integrity and generalization.
- Undervalued BI and reporting tools are struggling.
- Data management and security get complicated with multiple challengers.
A data warehouse is a powerful tool that helps companies analyze and use their data in a meaningful manner.
Data warehouses became such because this is how centralized databases were originally designed for analytical processing and decision support. Multiple data sources getting integrated are not the only things; the data go through transformation and structuring mechanisms optimized for the best querying and reporting conditions.
Key characteristics of data warehouses:
Schema-on-Write:
Data is processed during Extract, Transform, Load (ETL) steps, the quality of the data is kept stable while in the process.
Optimized for Analytics:
Consisting of various data nodes and archives for quick access to any required information, this technique of data warehouse exploits techniques like indexing, partitioning, and a columnar type of storage.
Data Integrity:
To achieve the quality and consistency of the data, there is a stricter level of data governance and quality controls.
Integration with BI Tools:
Data warehousing is equipped to break through BI and ordinary reporting thoroughly and fundamentally.
Pros:
- Firstly, database optimization helps with fast querying and reporting.
- Assures that the quality and integrity of the date are kept intact.
- Clings together with legacy BI tools tight together.
- Forged into the system that organizes and conducts data analysis and decision support.
Cons:
- Lack of the needed Flexibility to tackle unstructured or semi structured data problems could be a critical challenge.
- The scalability barriers to enormous data accumulation in spite of growing data volumes.
- Having a large amount of data integrated and maintaining this complexity.
You Need to Pick the Right Strategy for the Success of your Business.
Whether your organization prefers a data lake or data warehouse, or combination of both, is ultimately determined by your company’s unique needs, data needs, and analytical goals.Consider the following factors:
Data Diversity:
Data lake is the best storage option for the whole spectrum of structured, semistructured, and unstructured data for your organization if it deals with a lot of data points.
Analytical Requirements:
If your key concentration is conventional BI, reporting, and structured data analysis, then you can probably have a data warehouse, which may provide better integration and performance than other traditional tools
Scalability and Growth:
While a data lake can be a good choice for companies with a rapidly growing volume of data or need to perform analytics on big data, a data warehouse can be more cost-effective and offer flexibility.
Data Governance and Quality:
If strict data governance, quality controls, and consistency are critical for your business, a data warehouse may be the better option, ensuring data integrity and reliability.
Combining different strategies and the disruptive influence on the future are the topics to discuss
With data platforms continuing to transform and more and more organizations looking at hybrid approaches that take advantage of the best of both data lakes and data warehouses, we believe this phase is just the beginning.
These hybrid approaches exploit the extensibility, flexibility, and scalability of data lakes, as well as the capability to perform and handle structured data that data warehouses provide.
Future trends in data storage and analytics include:Future trends in data storage and analytics include:
Cloud-Based Solutions:
The cloud allows many organizations the flexibility and economies of scale to gain access to vast amounts of data storage independently from their infrastructure. In addition, cloud service providers are able to deploy both data lakes and data warehouses, with advanced analytics capabilities and scalable power.
Automated Data Management:
The new generation of technologies, including automated data consumption, processing, and governance, are helping to fight against data management problems through a reduction of manual work and an increase in quickness.
Real-time Analytics:
The rampant belief is that their data is a pivotal source of competitive advantage and the key to new product innovation & smart business decisions.
Integrated Platforms:
Nowadays, existing platforms provide integrated platforms with built in mechanisms that combine data lakes and data warehouses into unified platforms that are capable of catering to the needs of different storage and analysis areas.
Conclusion:
In the data driven world of 2024, putting the data lake or the data warehouse in place will depend on your organization’s particular needs in analysis and the growth of your future organization. As for data lakes, they allow a high degree of flexibility of data, scalability of processing, and integration with advanced analytics, but data warehouses are much more efficient in terms of performance, data integrity, and compatibility with traditional BI tools.
Thus, the best of the two would be the hybrid solution, which would capitalize on the strengths of both and make the realization of the full potential of our data achievable.
By effectively assessing the needs of your data and actively monitoring the progress of change in the field of data analytics, you can construct a strong data architecture that ensures that your institution is on the path to success with the popular trend changes in data analysis.
About the Author

Published by
BluePi
Data-Driven Business Transformation

Published by
Divya Dass
A data-driven solutions architect, leverages his expertise in data science, data lake management, data warehousing, and cloud CDPs to lead impactful data projects across diverse domains. A skilled communicator and collaborator, Divya translates data insights into actionable business strategies, continuously evolving and optimizing data-driven operations within the company.
Contact Us
RELATED BLOGS